Please select your location and preferred language where available.
We took on an unprecedented project to create manga characters of Tezuka with AI.
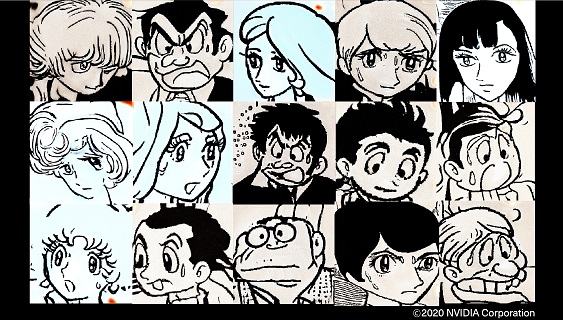
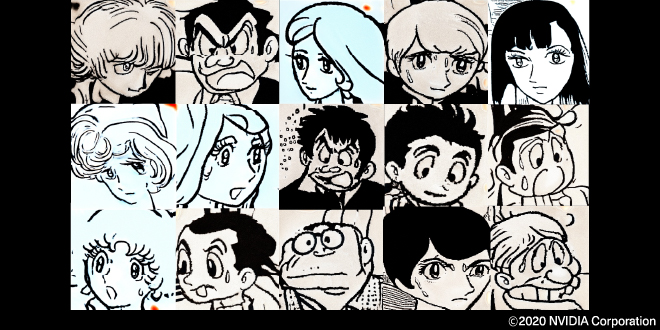
- TEZUKA2020 VOL.4 Character -
February 26, 2020
The AI that had learned 150,000 pages of manuscript data from Osamu Tezuka gave life to new characters. We spoke with creators, researchers and engineers who outfaced this grand project, “TEZUKA 2020”, and realized results after much trial and error.
The day when AI becomes a true partner will come.
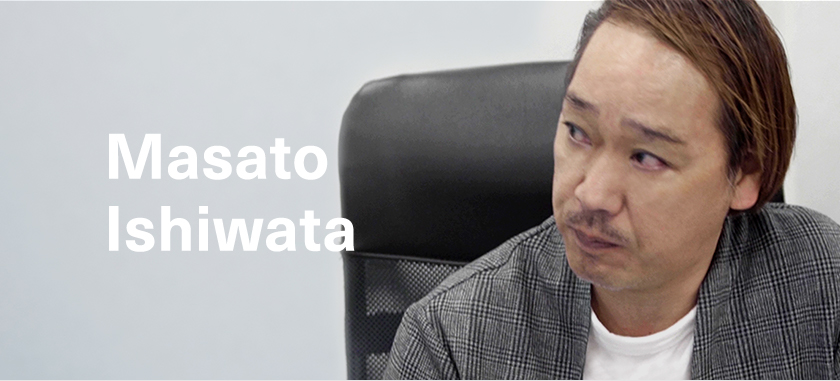
Director of Creative Division, Tezuka Productions
The diversity of these images makes it seem as though they weren’t drawn by a single artist. More than anything, I think that’s what “Tezuka-like” means.
Next, we speak with Masato Ishiwata who has been involved in “TEZUKA 2020” as a project leader and representative of Tezuka Productions. How did this project appear to Mr. Ishiwata, who played a key role in keeping the plans on schedule, from providing materials for AI learning to negotiating with publishers and producing manga art?
“Humans and AI working together to draw manga. At first, I was astonished by the high energy of the team on this still-undeveloped project. Then, I started to feel that we would usher in a new era and I became very enthusiastic about it myself.”
When I asked Mr. Ishiwata about the characters he felt were most Osamu Tezuka-like, he gave a surprising answer.
“Actually, when I’m asked what is ‘Tezuka-like,’ I find it a little difficult. Tezuka wrote and drew non-stop for 43 years after his debut in 1946, and his published works ranged widely from kids’ magazines to general magazines. He also responded to requests for sketches, patterns, and collective projects depicting famous characters, but the line thickness and touch, the proportions, etc. are so different in every instance that his work doesn’t appear to be the work of a single artist.”
“There was a different Osamu Tezuka for each era, genre, and readership,” Ishiwata continued.
“Even Astro Boy—he’s a robot, so his body never grows, but his proportions changed over the course of the 17-year series. In a previous interview, Osamu Tezuka mentioned how he changed Astro Boy’s head and body to keep up with the changing times. But no matter the era, the beauty of the lines depicting the character remains the same. Whether the line is straight or curved, they extend to the final point without hesitation, with a sense of speed. This was Osamu Tezuka’s most distinctive strength.”
At one point he was able to catch a glimpse of this project firsthand. Just as Astro Boy hoped that humans and robots could coexist, what if humans and AI could draw cartoons, each complementing the other’s strengths? When I imagined this, the future seemed even more interesting.
“People have a lot of different opinions about AI. But I think if we can learn to get along, we’ll be able to create better works of art. So I’m looking forward to the future. The day when AI becomes a true partner. I’m waiting for that day to arrive.”
Masato Ishiwata
Director of Creative Division, Tezuka Productions
After working for an advertising firm, Ishiwata joined Tezuka Productions in 2000. In addition to serving as Director of the Creative Division, he is also a visiting professor at Tokyo Fuji University, a visiting researcher at the Waseda University Media Culture Research Institute, and Vice Chairman of the Astro Currency Executive Committee, among other roles. His favorite Osamu Tezuka works are “The Amazing 3” and “Phoenix.” He has authored “The Robot Chronicles,” “Local Currency,” “Exploring Media’s Future,” and “Community Design with Astro Currency” (all in Japanese).
Development into co-creative collaboration between AI and humans
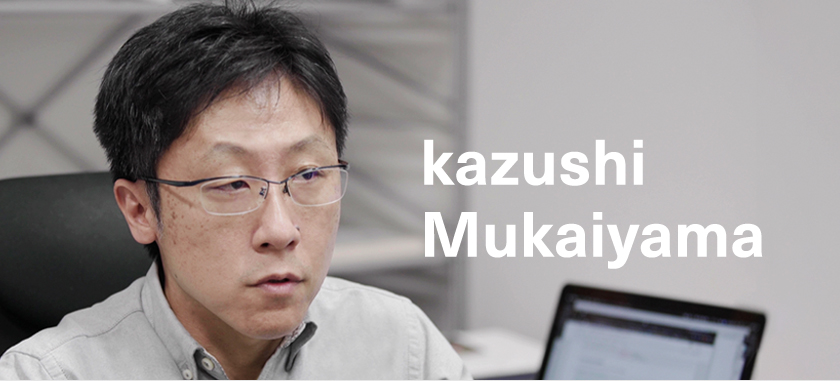
Professor, Future University Hakodate
The faces of the characters drawn by Osamu Tezuka are not recognized as faces by AI.
Professor Mukaiyama is conducting research at Future University Hakodate in which he has computers draw pictures, instead of humans.
“As I pursue my research, I find there are human activities that computers are incapable of replacing. These are ‘creative things’ that only humans can do, and at present, I continue to research to find out what these things are.”
Professor Mukaiyama’s task was to extract frames and speech balloons from Osamu Tezuka manuscript data he received from Tezuka Productions using a recognition software and have the AI learn it.
“In order for AI to perform machine learning, we must first teach it what a face is. In addition, the characters drawn by Tezuka-sensei are somewhat distorted, so the recognition software we initially used to identify human faces wasn’t up to the task. As a result, we decided to select images that we could use, but it wasn’t just one or two—we might need 10,000 images of faces in some extreme cases. I started by gathering these images together with my students.”
This task was incredibly difficult. First, he decided to extract facial images one by one from works such as “Black Jack,” “Phoenix,” and “Astro Boy”, but it took a tremendous amount of time. After this process came to end, he then started tackling other works.
“I struggled with the software’s lack of flexibility,” he laughed. “I repeated a process of trial and error—I had the computer learn character faces outside of Tezuka’s works as well as actual human faces, and I made it learn the augmented data generated by flipping the characters’ faces left and right.”
Professor Mukaiyama has been tackling this challenge from various angles to improve the precision of AI’s ability to categorize images. He offered the following comments on his impressions of participating in this project.
“Even if AI can learn to draw manga art in the future, we shouldn’t expect human creativity to fall to zero. This is because manga has readers as well as creators. Looking at the history of manga so far, new onomatopoeia and speech balloon expressions have been created, but these don’t take root unless readers accept them. I feel that the same thing will happen in this project. How large a role will AI play? How large a role will humans play? I think that standards for addressing this will emerge someday. It might be in 10,000 years from now, but I’m looking forward to seeing how human creativity turns out.”
Kazushi Mukaiyama
Professor, Future University Hakodate
Graduated from the Kyoto City University of Arts doctoral program in media arts. From 1998, he was a visiting artist for two years at UC San Diego, at the Institute of Computing (now the Arthur C. Clarke Center). In 2000, he won the Prix Ars Electronica in the .net category. In 2011, he was chosen for Sao Paulo FILE Festival 2011. In 2016, he was IMAC guest lecturer at Université Paris-Est Marne-la-Vallée. His interests lie in information processing for creative activities, and he researches and publishes on human cognitive traits by tasking artificial intelligence to draw pictures. When he was a child, he learned kanji characters by reading “Astro Boy” manga.
A continuing process of trial and error for character creation in the unprecedented challenge
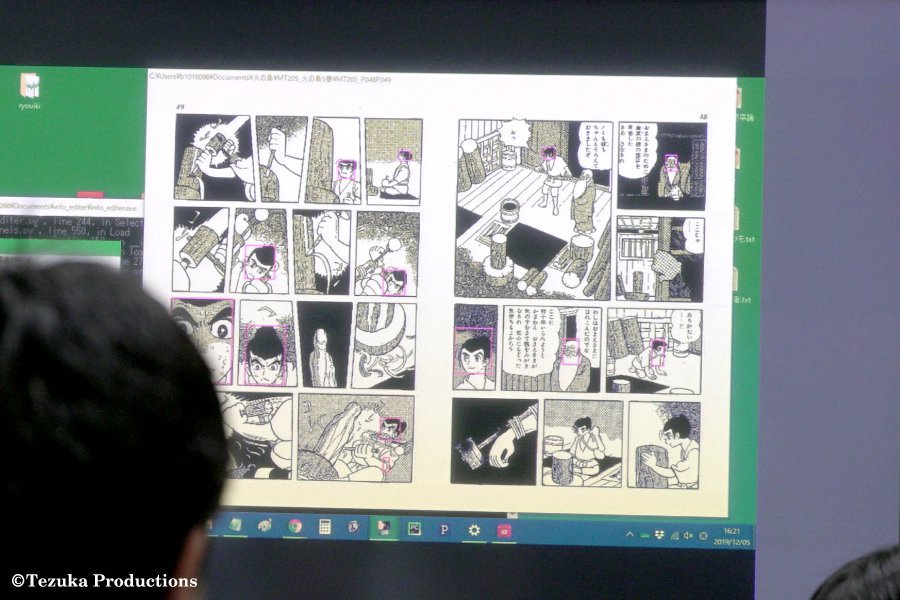
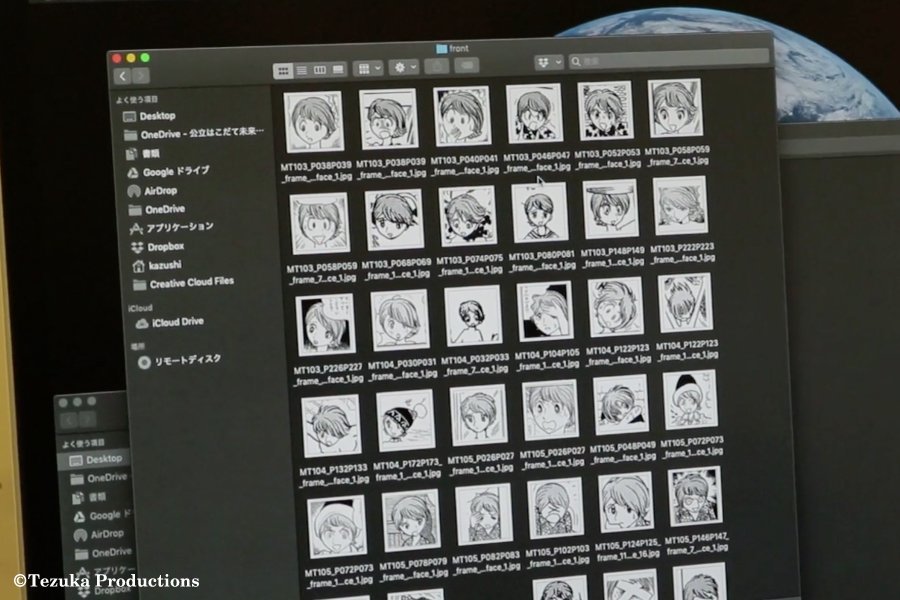
150,000 pages of manuscript data from Osamu Tezuka were prepared for the AI to learn what “Tezuka-like” characters are. These were passed through the recognition software at Future University Hakodate, and were classified and tagged as “frames,” “speech balloons,” “faces,” and “bodies.” To improve the quality of the images generated by AI, the staff repeated a process of trial and error until the ideal images were generated—for example, by flipping, then increasing the number of images recognized, loading only female characters, and so on.
A breakthrough for character generation by transfer learning
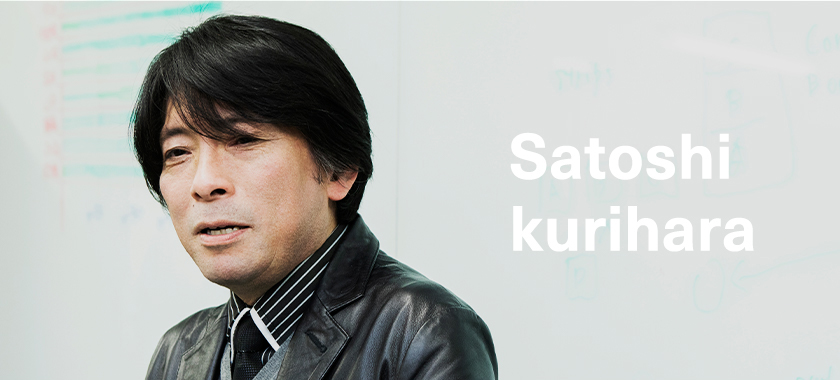
Professor at the Faculty of Science and Technology, Keio University / Specially-Appointed Professor at the Artificial Intelligence eXploration Research Center, The University of Electro-Communications
The first attempt was generating monochrome manga images with AI.
This presented us with a series of unknown challenges.
“The AI we used for image generation can be taught the features of ‘faces’ by feeding a huge number of facial images, and it is capable of generating extremely realistic human faces that don’t actually exist. At first, we tried to train the AI simply by giving characters drawn by Osamu Tezuka alone. Our effort to have an AI generate monochrome manga line drawings was perhaps the first attempt ever. This was unknown territory for us, too.”
As far as the outcome is concerned, the characters were not generated as expected but Prof. Kurihara suggested that this was to be expected.
“I didn’t think we’d be able to generate beautiful facial images from the very start. However, although we generated something like a face but not quite a face, I think it certainly had a “Tezuka-like” quality to it. At first, I thought the next goal might be for humans to finish it off and turn it into a character.”
There was a limit to learning “Osamu Tezuka-like” qualities in this approach, because front-facing images of characters are extremely rare in manga.
“But since the AI we’re using is capable of generating quite high-quality realistic human faces, we’d feel ashamed if we couldn’t create something that looked a little more like a face...” he laughed.
“So, with help from Kioxia, we decided to use the transfer learning technique to utilize the facial learning acquired by an AI that has already learned from the data of hundreds of thousands of faces.”
This concept brought the project back on track, but there was a long way to go.
“There were a lot of twists and turns and trial and error that led to our use of the transfer learning technique—it was an interesting development path, I feel.”
Satoshi Kurihara
Professor at the Faculty of Science and Technology, Keio University / Specially-Appointed Professor at the Artificial Intelligence eXploration Research Center, The University of Electro-Communications
After graduating from the Graduate School of Science and Technology at Keio University, he worked first for NTT Basic Research Laboratories, and then later as an Associate Professor at The Institute of Scientific and Industrial Research at Osaka University and as a Professor at the Graduate School of Informatics and Engineering, before moving into his current role. He has doctorate degree in engineering. His favorite works by Osamu Tezuka are “Jungle Emperor Leo”, “Princess Knight”, and “Black Jack”. He has written many books, including “Artificial Intelligence and Society” (Ohmsha, Ltd) and edited “Encyclopedia of Artificial Intelligence Studies” (Kyoritsu Publication). His latest work is “AI Weapons and Society in the Future: the True Identity of Killer Robots” (Asahi Shinsho).
What is GAN deep learning, which produces characters as close as possible to Osamu Tezuka’s?
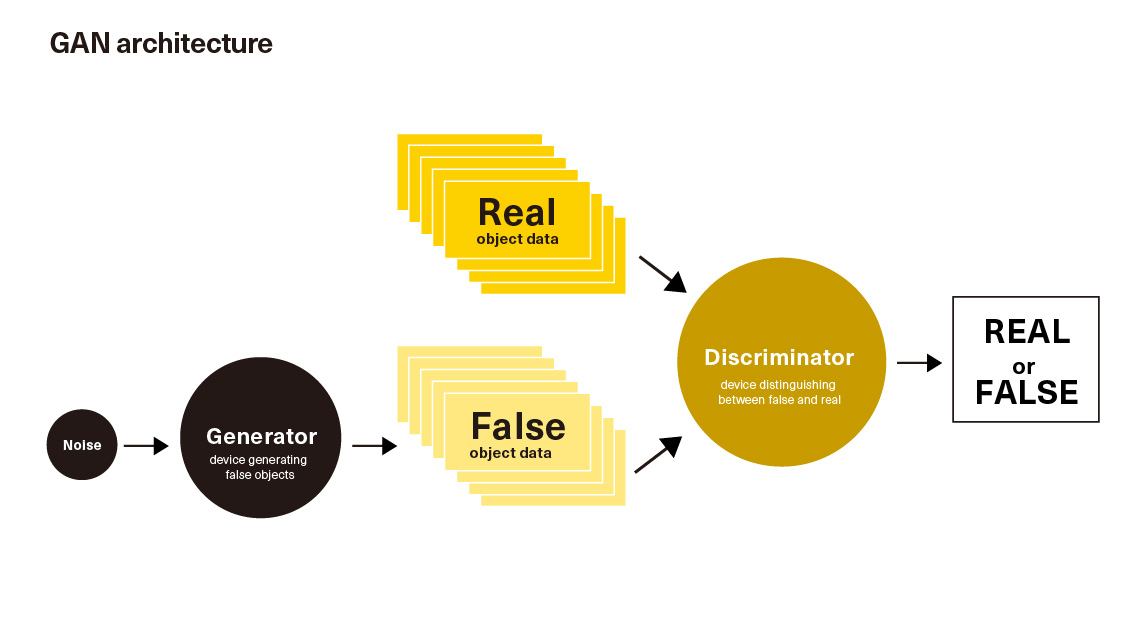
At the core of character generation is an AI technology called GAN (Generative Adversarial Networks), which is capable of generating images as close to real as possible by learning what “realness” is.
GAN consists of two networks. The learning process itself is “adversarial” in that one network, the discriminator, identifies whether or not the generated data is close to the input real data, and another network, the generator, generates higher quality fake data so that the discriminator cannot distinguish it from the real data.
By a process of repeated generation and discrimination, the generator learn how to produce data similar to the real data. Although this system was originally used at Kioxia to improve semiconductor design and manufacturing quality, it was applied to character generation for this project.
However, the challenges of AI mean it isn’t an easy process, which is what makes it interesting. For “TEZUKA 2020”, the GAN system that studied “Tezuka-like” properties was further incorporated into two other types of AI technologies to attempt character generation. This resulted in the following three systems.
GAN (1)
In the initially proposed GAN, an image of the entire face was generated in a single process, so the details were not fully complete. For this reason, the team attempted to generate more complete images using a new GAN method where fine details such as the eyes, nose, and mouth are gradually generated from rough depictions, such as contours. Image-like generation results were obtained after initially training on about 4,500 images, but later, the learned images were flipped left and right. By training on the flipped data, the system learned about 18,000 pieces of data—about 4 times as many. This is a major step forward, because the more training data, the better the accuracy.
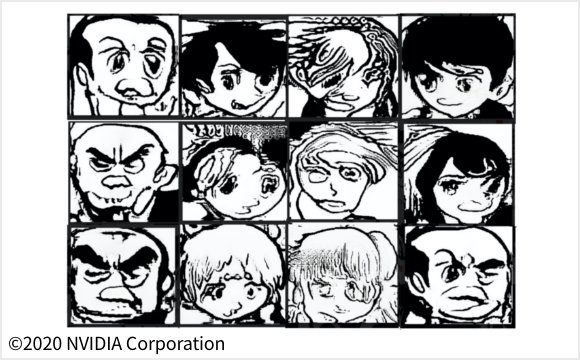
GAN (2)
However, it was still far from ideal. Next, the team decided to use only female characters from Tezuka’s manga and start learning from scratch. The results were quite good, probably because the female characters had relatively well-designed patterns in many cases. After that, various other approaches were tried, such as learning by mixing character images.
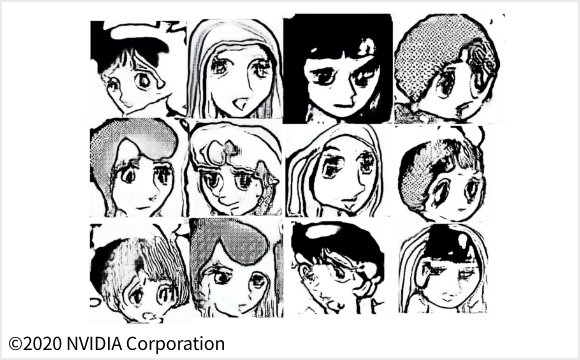
GAN (3)
Ultimately, the decision was made to introduce a technology called “Transfer learning.” Incorporating hundreds of thousands of data points, the AI learned what human facial features are from an AI that had already learned this, then the AI studied Tezuka’s manga characters as additional learning. This enabled the generation of “Tezuka-like” characters without fail, and the project started to make great progress. “PHAEDO” emerged from this process.
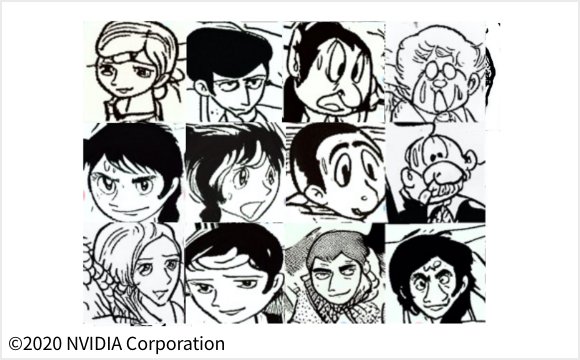
The determination of a development professional drives the project forward.
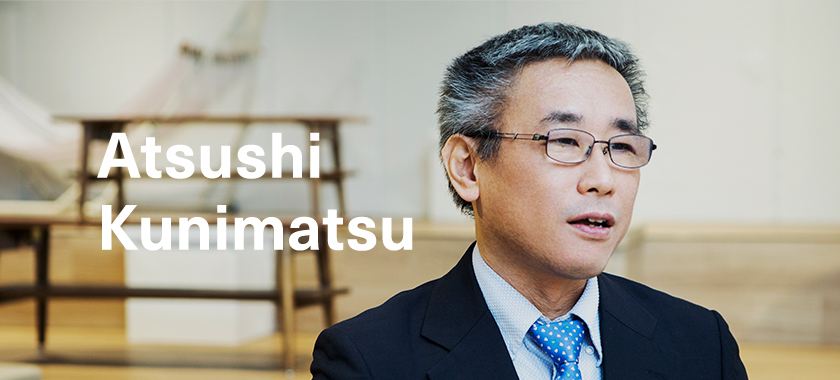
KIOXIA Corporation cSSD Engineering Department
The key to moving the project forward was transfer learning using real human faces for the AI to study
The early AI learning about manga characters wasn’t able to generate characters the way the team had expected. Although the project team gave repeated feedback to the AI and made constant improvements, but the real breakthrough was a shift in approach to introduce transfer learning. So what exactly is transfer learning?
Atsushi Kunimatsu at KIOXIA, an advocate to employ this technology notes, “In a nutshell, it’s a two-step learning process. At first, the AI started learning using only Tezuka’s manga art—this was a one-stage learning process. However, because manga art is full of two-dimensional human faces, we decided to have the AI first learn about the structure of human faces first, using images of real human faces, then learn about Tezuka’s manga art.”
“The bigger the project, the more likely that the unexpected will occur. That’s why it’s important to have backup plans—Plan B and Plan C—separate from the main plan. Maybe those backup efforts would never come to fruition. However, we were taking on a first-ever challenge in the world, so it was only natural. Of course, if we over-insured ourselves with backups and went broke as a result, we’d have our priorities backwards,” he laughed. “At Kioxia, we’re development professionals. This is it’s so deeply engrained for us to think about backup plans for when things go wrong.”
With the introduction of transfer learning, “Tezuka-like” characters can now be generated, which moves the project forward by leaps and bounds.
Atsushi Kunimatsu
KIOXIA Corporation cSSD Engineering Department
He’s taking part in “TEZUKA 2020” as an engineer at Kioxia. He took a lead role in the project, coming up with ideas for character generation technology. He usually works on SSD (flash memory) development. He first encountered Tezuka at elementary school, where he read a lot of Tezuka’s works at the library, including “Black Jack” and “Kimba the White Lion.” His favorite was “The Three-Eyed One,” due to his love of ancient archeological sites.
What is “transfer learning,” the key to problem-solving here?
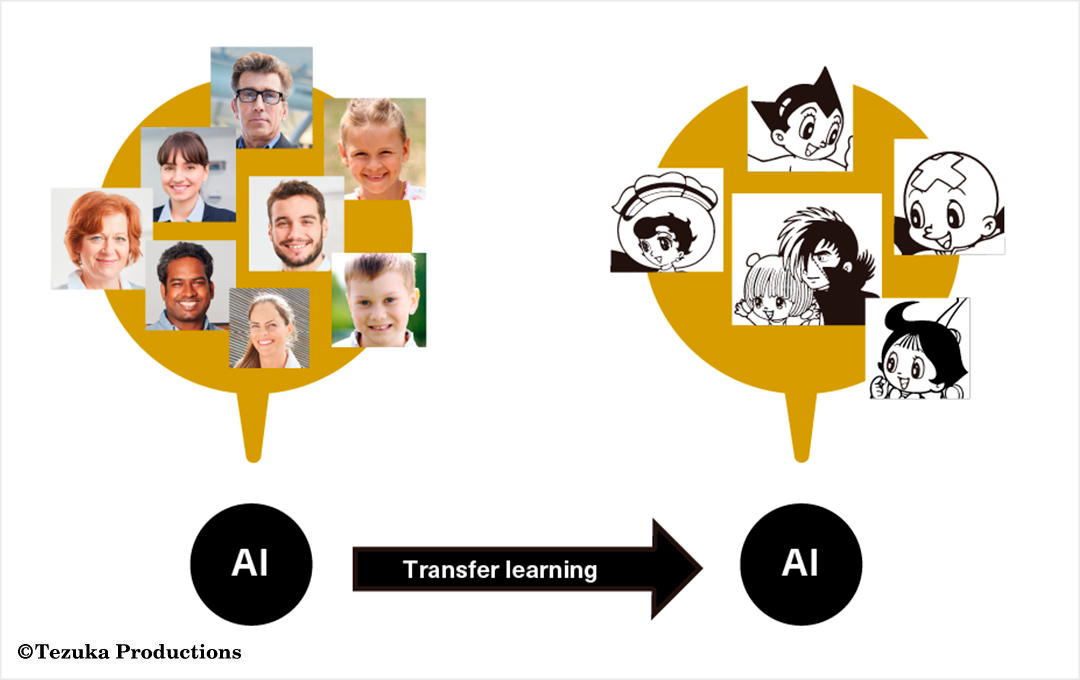
Transfer learning is a technique for adapting a model that has been trained in one domain to another domain. This may seem like a difficult concept, but when applied to this project, it involves adapting “a model that has learned the structure of human faces” to “a model that has learned Tezuka’s manga art style” (additional learning).
In the initial attempts, the team tried to create new characters by letting an AI learn Tezuka’s manga characters alone, but with the transfer learning technique, an AI already capable of generating human faces is tasked with learning about Tezuka’s manga art characters.
Essentially, with an AI, the larger the amount of data learned, the higher the quality of the data it generates. Using Tezuka’s manga comics alone, the limit was some tens of thousands of images, but using actual human facial images expanded the AI learning to several hundreds of thousands of data. The key to success was a dramatic increase in learning using transfer learning.
The last challenge that resulted in improving the precision of characters
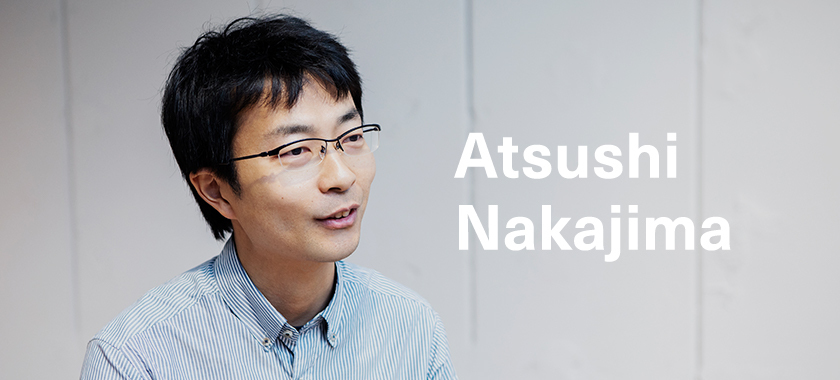
KIOXIA Corporation, Institute of Memory Technology Research Development, System Technology R&D Center
Combining multiple individual features to create more original characters. It feels like we’re looking at a futuristic manga right here.
Transfer learning helped the stalled project move forward again. How did this idea, the turning point of the project, actually arise? And what other challenges were attempted after this? We spoke with Atsushi Nakajima from Kioxia, who was in charge of this process.
“The transfer learning was working well, but there were still issues."
The question was whether it was generating characters that suited the story. The outline of the story had already been determined and they were looking for a character capable of strongly conveying emotion with his or her eyes, but it wasn’t so easy to create the ideal character.
“That’s when we tried the method of combining the individual features of the characters born out of transfer learning. Using this method enabled us to map the characteristics of one character onto a different character. For example, letting the eyes of one character onto the eyes of another character, or combining two characters together then separating them to create an intermediary character”
All the basic ideas for the main characters appearing in “PHAEDO” were created by AI. Mr. Nakajima has stated that it gave him a glimpse into the future of manga comics.
“I don’t know when, but I’m sure that in the future, manga will be successfully produced by AIs alone. For example, if you don’t like the story’s development, you might create a new story together with the AI. Creating this sort of manga may become commonplace.”
Atsushi Nakajima
KIOXIA Corporation, Institute of Memory Technology Research Development, System Technology R&D Center
He reached his current position after developing TVs and video recorders. He has applied machine learning to improve the efficiency of production and design, and to improve learning efficiency, especially in the field of image generation. For “TEZUKA 2020”, he implemented the idea of transfer learning. He was part of the generation that learned about the existence of Osamu Tezuka from elementary school textbooks. He enjoyed the anime version of “The Three-Eyed One,” which he watched as a child, and was thrilled with the story’s message: “When you face difficulties, try to work it out and solve the problem yourself.”
Mixing different individual features enabled the generation of more suitable characters for the story.
By combining different individual features of the characters, we found that characters with unprecedented characteristics could be created. This mechanism relies on creating a number of variations by gradually changing the ratio of the two characters that are mixed. Here’s what it actually looks like, along with an animation.
©2020 NVIDIA Corporation
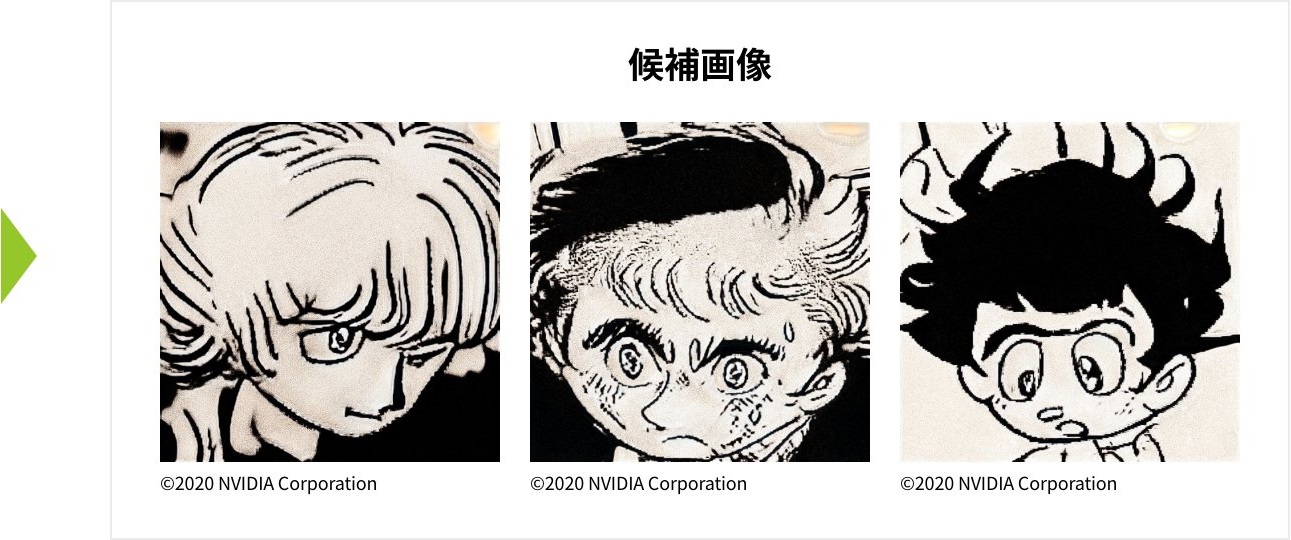
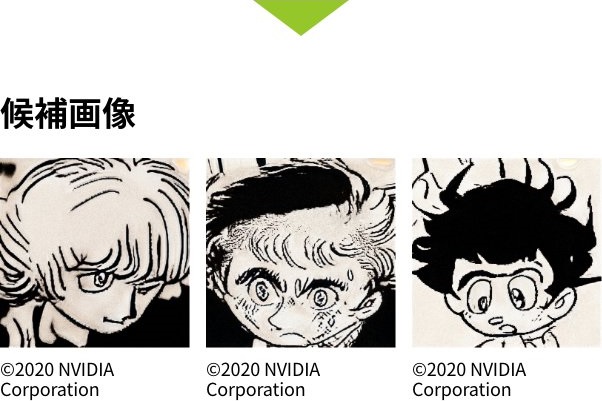
Here is an image extracted from character we generated. You can see that the details of the characteristics change while the overall atmosphere is maintained.
The content and profile are current as of the time of the interview (February 2020).