Please select your location and preferred language where available.
Kioxia Presented Image Classification System Deploying Memory-Centric AI with High-capacity Storage at ECCV 2022
- November 2, 2022
- Kioxia Corporation
Tokyo - Kioxia Corporation, the world leader in memory solutions, has developed an image classification system based on Memory-Centric AI, an AI technology that utilizes high-capacity storage. The system classifies images using a neural network that refers to knowledge stored in external high-capacity storage; this avoids "catastrophic forgetting," one of the major challenges of neural networks, and allows knowledge to be added or updated without the loss of current knowledge. This technology was presented on October 25 at the oral session of European Conference on Computer Vision 2022 (ECCV 2022) in Tel Aviv, one of the top conferences in the field of computer vision*1.
In conventional AI techniques, neural networks are trained to acquire knowledge by updating parameters called “weights.” Once fully trained, in order to acquire new knowledge a neural network must be either re-trained from the beginning or fine-tuned with new data. The former requires huge amounts of time and consumes significant energy costs, while the latter requires parameters to be updated and faces the catastrophic forgetting problem of losing the knowledge acquired in the past which leads to deterioration of classification accuracy.
To address the issues of cost and accuracy in neural network-based image classification systems, the new solution stores large amounts of image data, labels and image feature maps*2 as knowledge in a high-capacity storage. The neural network then classifies images by referring to that stored knowledge (Figure 1). Using this method, knowledge can be added or updated by adding newly obtained image labels and feature maps to the stored data. As there is no need to re-train or update weights, which may cause “catastrophic forgetting,” image classification can be maintained more accurately.
Furthermore, by using the data referred from the storage when the neural network classifies images, the basis for the classification results can be visualized, which is expected to improve the explainability of AI*3 and alleviate the black-box problem*4, in turn allowing the selective modification of knowledge sources. In addition, by analyzing the referred data, the contribution of each stored data can be evaluated according to the frequency of references.
Guided by its mission of "Uplifting the World with ‘Memory,’" Kioxia will continue to contribute to the development of AI and storage technologies by expanding Memory-Centric AI beyond image classification to other areas and promoting research and development of AI technology utilizing high-capacity storage.
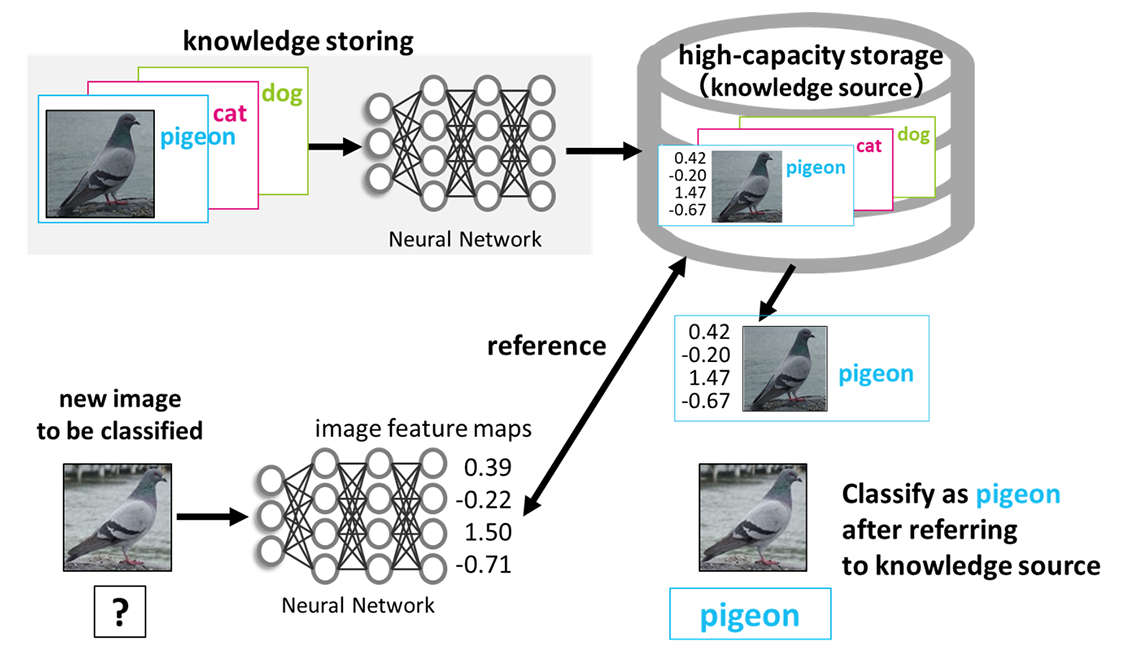
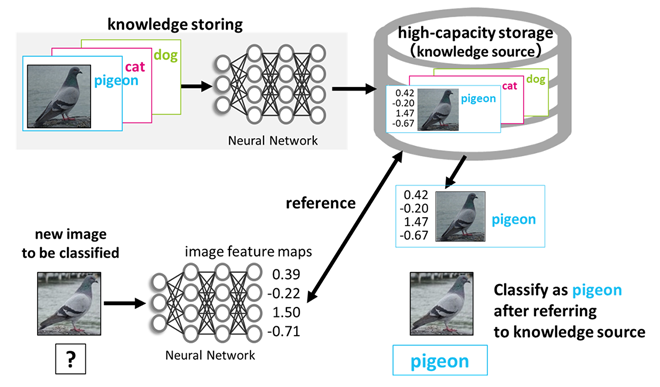
Figure 1 - Image classification utilizing high-capacity storage
About Memory-Centric AI
- Introductory Video: https://youtu.be/lw8XKhviGJc
- Memory-Centric AI, Part I: How Kioxia’s Top Engineers Are Developing an AI That Relies on Memory
https://brand.kioxia.com/en-jp/articles/article25.html - Memory-Centric AI, Part II: An Internet of Memories: Brainstorming Uses for Memory-Centric AI
https://brand.kioxia.com/en-jp/articles/article26.html
About ECCV
The European Conference on Computer Vision (ECCV) is one of the top conferences in the field of computer vision. In recent years, ECCV has established itself as a prime venue for the presentation of AI research papers including image classification, object detection, and other technologies using deep learning. The oral presentation acceptance rate was 2.7% in this year.
- Paper title: K. Nakata et. al., “Revisiting a kNN-based Image Classification System with High-capacity Storage”, European Conference on Computer Vision 2022 (ECCV 2022)
- Image feature maps: multidimensional (e.g., 1,024-dimensional) numerical data obtained through neural network operations
- Explainability of AI: possibly of explaining the basis and reasons of results predicted by AI in a way that can be interpreted by humans.
- Black-box problem: the process leading to the results predicted by AI is not interpretable to humans, making it a black box problem.
- Company names, product names, and service names may be trademarks of third-party companies.
Information in this document, including product prices and specifications, content of services and contact information, is correct on the date of the announcement but is subject to change without prior notice.